It’s now widely acknowledged that artificial intelligence has made rapid progress in recent years. Many applications of AI are now outperforming humans at specific tasks – such as game playing and diagnostic systems. Most of this has been achieved within the last decade through rapid progress using data-driven approaches that are centered on machine learning technologies and algorithms. However, despite all the euphoria, many AI researchers believe that machine learning alone is not enough to produce human-level intelligence. Human-level intelligence has come to be known as Strong AI or Artificial General Intelligence (AGI).
Humans have the ability to gain and apply general knowledge to problem solving for a wide range of subject domains. Some of which is acquired by all human beings, such as walking and talking. Some human beings acquire specialist knowledge, usually as part of their vocations, such as medical surgeons, civil engineers, or lorry drivers. Having general intelligence enables us to combine broad cognitive abilities and to flip seamlessly from one knowledge task to another when required to facilitate problem solving.

However, despite the phenomenal progress in AI, AGI is still seen as some way off fulfillment. In this article, I briefly explain why. In a follow up article, I will describe the pathways that may make it happen in the future.
The Current AI paradigm – deep learning
AI has made a huge impact in the last five years. Hardly a day passes without AI applications getting media coverage and start up activity has soared with business start-ups booming in this field. For example, according to this report AI related companies saw a 72% increase in activity in 2018, compared with 2017.
Machine learning, a branch of AI, has been the catalyst for this increased activity. This term refers to methods that enable the machine to learn without being explicitly programmed. The main paradigm used in machine learning has been Artificial Neural Networks (ANNs). The concepts of ANNs have been used experimentally since the 1960’s, and owe their origin to the operation of neurons in the human brain, but in the last decade they have come of age through a neural network architecture called deep learning. Such a network consists of a hierarchy of layers, called hidden ayers, such that each layer can help identify more abstract representations of patterns being investigated. Using this approach, they can be used to recognize patterns in the same way that we human beings can identify, such as people’s faces, and so on. This has made an impact because the early ANN’s were based on perceptron software that used only single layers of neurons and lacked access to large amounts of data needed to train systems to accomplish these tasks. However, deep learning algorithms use multiple layers of ANN’s combined with humungous amounts of data – now available though social networking and commerce sites on the World Wide Web. They improve their performance by fine tuning parameters received from sensory inputs from their environment.
Deep learning began making a big impact in 2012, through the use of a successful pattern matching paradigm in image identification. Its success has been extended since then in other domains. Applications using deep learning are being used extensively in business, commerce, and many other domains, such as healthcare. As the algorithms improve and the hardware gets more powerful, many new applications will emerge and AI will become ubiquitous – if it isn’t already. There are many specific applications of deep learning that now outperform humans. For example, AI chess surpassed humans some time ago. But for Go, a very complex game that has its origins in China, it was thought unlikely that AI would beat grandmasters for many years to come. However, an AI program, called AlphaGo, developed by Google DeepMind, beat the reigning human champion in 2016. It achieved this by by studying the moves of human experts and by playing against itself many, many, times. In effect, it was its own teacher using a paradigm called reinforcement learning. This is a type of learning that learns from its own previous actions when playing against itself.
The shortcomings of deep learning
Despite the phenomenal success of Deep Learning AI, Some experts question whether this paradigm is sufficient for human-level intelligence. For example, Francois Chollet, a prominent researcher of deep learning networks, believes that you cannot achieve general intelligence simply by scaling up today’s deep learning techniques.
There are also other challenges for this technology. One of the shortcomings of ANN’s is that they are woefully inadequate in explaining and making transparent their decision making reasoning. They are black box architectures. This is particularly problematic in applications such as healthcare diagnostic systems — where practitioners need to understand their decision making processes. As J. Brockman [1] says, the reason why ANN’s lack transparency is that: “they operate in a statistical, or model-blind mode, which is roughly analogous to fitting a function to a cloud of data points”. This is not surprising, because they are data-driven statistical number crunchers they have little causal understanding of what is going on. This means that there is no trace back facility for causal reasoning and therefore they cannot serve as a model for human level AI. Understanding the reasoning behind decisions can form the basis for explanations such as: Why is the system asking for input information, or “how did the system arrive at its conclusions”. Explanations are considered very important components of AI improvements. So much so that DARPA (Defense Advanced Research Projects Agency), has for some time regarded the current generation of AI technologies as important in the future but regard their black box nature as being a severe impediment to the use of the technology. DARPA is a division of the American Defense Department that investigates new technologies. It is against this background that DARPA announced, in August 2016, that they would provide funding a series of new projects, called Explainable Artificial Intelligence (XAI). They say that the purpose of these projects is to create tools that will enable a human on the receiving end of a decision from an AI program to understand the reasoning behind that decision. I have already written about Improving transparency in AI programs importance, because, deep learning systems can sometimes make unpredictable decisions and trust in these systems is therefore, crucial to their acceptance.
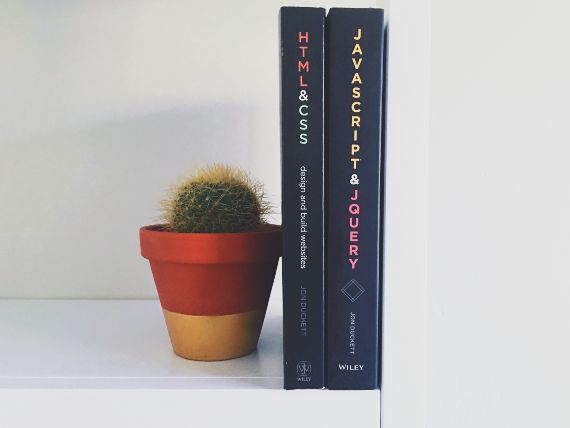
It may seem surprising to note that the older, previous generations of AI, known as GOFAI (Good Old Fashioned AI), did have some limited capabilities for explanation because of the explicit way that their knowledge was represented and manipulated. But GOFAI systems had very limited learning capabilities. Some GOFAI systems work well, and still do today. But their inability to learn became a severe impediment to their acceptance. Without learning capabilities, they could not adapt to changes in their environment that is a crucial component of self-driving cars, image recognition, robotics, and other AI learning systems.
Is deep learning enough to deliver AGI?
There are some encouraging signs on the horizon. For example, Demis Hassabis of DeepMind , has stated that he believes the key to AGI lies in what he calls transfer learning. This is a technique whereby a model trained on one task is re-purposed on a second related task. This technique is borrowed from humans when they sometimes learn new tasks. For example, research has shown that bilingual language speakers generally find it easier to learn new languages, because the precedent knowledge learned from becoming bilingual, can be re-applied and facilitate the learning process of new languages because they become aware of the need to learn structures and language syntax that speakers familiar with one language would not.
When applied to machine learning, this leads to the general belief that the precedent knowledge learned from one task, will help enable faster training and require less supervision than one trained from scratch on the second related task. However, for transfer learning to lead to AGI, it would have to be able to transfer the learning across a wide range of subject domains. As Hassabis says:”I think the key to doing transfer learning will be the acquisition of conceptual knowledge that is abstracted away from perceptual details of where you learned it from.” He also acknowledges it is still a big challenge for the AI community. As he says, “It works reasonably well when the tasks are closely related, but transfer learning becomes much more complex beyond that”.
Many senior practitioners thus, have doubts about AGI ever happening using deep learning alone. However, there are other possible pathways to AGI that I will discuss in my next article.
References
[1] Brockman, J. Possible Minds 25 ways of looking at AI. Chapter written by Pearl, J. 2019.
Keith Darlington
Comments on this publication