While at the beginning we tolerated the intrusion of Artificial Intelligence (AI) on issues that we generally give little importance to, such as suggesting a movie to watch on Netflix or prioritizing the content in our social networks, we are closer and closer to seeing it being used for decisions of greater depth, such as helping doctors in making their medical diagnoses.
AI has the ability to interpret and analyse a lot of information quickly, which is very promising in the field of medicine where more and more digital data is being generated. Tasks such as the development of new drugs, the sequencing of DNA, the use of implants and smart patches, the remote monitoring of patients and the carrying out of epidemiological studies with thousands of patients are some of the fields that could benefit from this technology in the near future.
But experts agree that one of the most fertile grounds to take advantage of AI in medicine is the acquisition and interpretation of images for diagnosis—such as ultrasound, computerized tomography (CT) or magnetic resonance imaging (MRI). The results, in the form of digital images, must be interpreted by the doctors, who with their training and expertise can extract useful information to reach a diagnosis.
As the number of images that are acquired—and their quality, sensitivity and resolution—increase steadily, researchers are working to develop technologies to help radiologists assess these images more quickly, accurately and effectively. There are numerous projects and lines of research under way to take AI into the radiology department.
Many applications
One example is the system developed by Francisco Albiol (IFIC/CSIC-University of Valencia) and his team, in which they have trained a computer program in the early detection of breast cancer. This work has been done as part of the Digital Mammography DREAM Challenge, a competition open to research groups from around the world. For this they have developed an algorithm that manages to detect breast cancer in mammograms with a precision of between 80% and 90%, a percentage of success similar to that of a doctor. If the computer method is combined with the expertise of the professional, the detection accuracy reaches up to 95%. Globally, this improvement could reduce the number of false positives in mammograms, thus preventing millions of women from having to undergo unnecessary biopsies.

Another example is the CheXNet project, developed at Stanford University (USA), based on deep learning—a method for the AI to learn by itself from large collections of data—to detect cases of pneumonia on radiographs. For this they have used more than 120,000 radiographs belonging to a database published by the National Institute of Health (USA). The result is that CheXNet is capable of exceeding human radiologists in accuracy. From the x-ray image it indicates the probability that the patient has pneumonia and identifies the areas of the image where the signs of it are most evident.
Also at Stanford, a group of engineers led by Sebastian Thrun (Stanford Artificial Intelligence Laboratory) have used the same technique to detect melanomas in images of skin lesions. Thrun and his team trained a computer using 130,000 images of lesions caused by 2,000 different pathologies. They compared the diagnoses made by their system with those from 21 dermatologists, achieving results equivalent to those obtained by the specialists. Currently Thrun and his team are working to modify their algorithm so that it can be used from a mobile phone, thus universalizing access to this technology.
Other initiatives include an algorithm developed at Harvard University (USA) that is capable of diagnosing chronic obstructive pulmonary disease from CT scans, or the iFind project led by King’s College London (United Kingdom), dedicated to developing an automated method to acquire and interpret pregnancy ultrasounds.
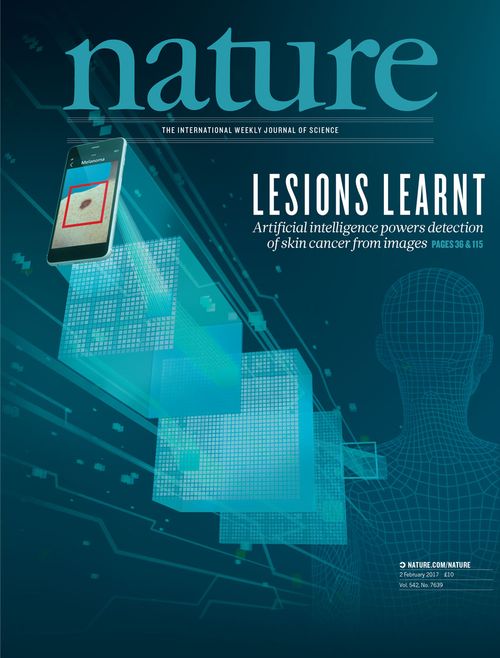
Pending challenges
However, there are still some details to work out before bringing AI-assisted medical images to the doctor’s office in a generalized manner. Access to large collections of data to train the algorithms generates problems ranging from those related to patient privacy to the management and storage of data. “Data is the new source of knowledge,” says Francisco Albiol. “For us it was fundamental to be able to access these collections, because it is not easy to get 800,000 images such as those we were able to analyse.” Another problem is that for the images to be used in the training of the algorithms, they must be correctly labelled with the diagnoses and the medical data of the patient.
Furthermore, there is the question of interpretability: knowing why the algorithm has reached one conclusion and not another. “The current neural networks are so complex that it’s impossible to know exactly what is happening,” explains Germán González, CEO of Sierra Research S.L., a consulting firm specialized in medical image analysis. “A modern neural network has millions of parameters and each one has its importance.” In addition, whenever new cases are added to the training of the neural network this changes its results slightly.

To reduce all these problems, a regulatory process is necessary to verify that the systems are reliable. In the United States, in order to use a method based on artificial intelligence in the clinical setting, it must be approved by the National Food and Drug Administration (FDA).
Future vision
As things stand now, although great progress is being made, it’ll still be awhile before we see the use of technologies based on deep learning fully entering the medical practice. According to González, one of the main factors will be the regulation of the sector. “There are cases in which I see clearly that it’s going to be applied,” he says, referring to support systems for the detection of pathologies in images. “For more advanced things like the diagnosis of a disease without a second review from a doctor, we are years away from that happening.”
For his part, Albiol emphasizes that this technology has great potential when looking at the big numbers, highlighting the fact that achieving an improvement of 5% or 10% in matters that affect millions of people every year has a great impact. “When the numbers are applied on a large scale, the number of beneficiaries is enormous,” he concludes.
Comments on this publication